EVOP-MS20
Evolutionary Theory of Disease
Thursday, June 17 at 11:30am (PDT)Thursday, June 17 at 07:30pm (BST)Friday, June 18 03:30am (KST)
Organizers:
Jesse Kreger (University of California, Irvine, United States), Natalia Komarova (University of California, Irvine, United States)
Description:
The use of fundamental principles of evolutionary biology can provide important insight into disease dynamics, both within and between hosts. For example, evolutionary mathematical models have been used to better understand disease progression, immune escape mechanisms/drug resistance, and optimal treatment regimens in many prominent diseases (such as virus infection and cancer). Our mini-symposium focuses on the “Evolutionary Theory of Disease”, and in particular, on how evolutionary ideas in tandem with mathematical techniques and models can be used to understand disease dynamics as well as how to best combat the disease. In our mini-symposium, researchers will present their exciting and impactful recent research, including new mathematical and computational models, with a focus on understanding the evolutionary dynamics of the disease. Our list of speakers includes both accomplished/senior researchers as well as junior mathematicians at the postdoctoral and graduate student levels. Their research spans different diseases (from infectious diseases to cancer) and mathematical approaches (including differential equations, agent-based models, network models, etc), and should stimulate broad interest in the mathematical biology community. We look forward to an exciting exchange of ideas, as well as great networking opportunities for researchers at all career stages.
Chadi M. Saad-Roy
(Princeton University, United States)"The evolution of an asymptomatic infectious stage: analysis of a simple evolutionary-epidemiological model"
Jasmine Foo
(University of Minnesota, United States)"Power law transitions in site frequency spectra of neutrally evolving tumors"
Mohammad Kohandel
(University of Waterloo, Canada)"Predicting mutability of the genomic segments of a pathogen"
Alison Hill
(Johns Hopkins University, Institute for Computational Medicine, United States)"Selection for SARS-CoV-2 variants at the within-host and population scale"
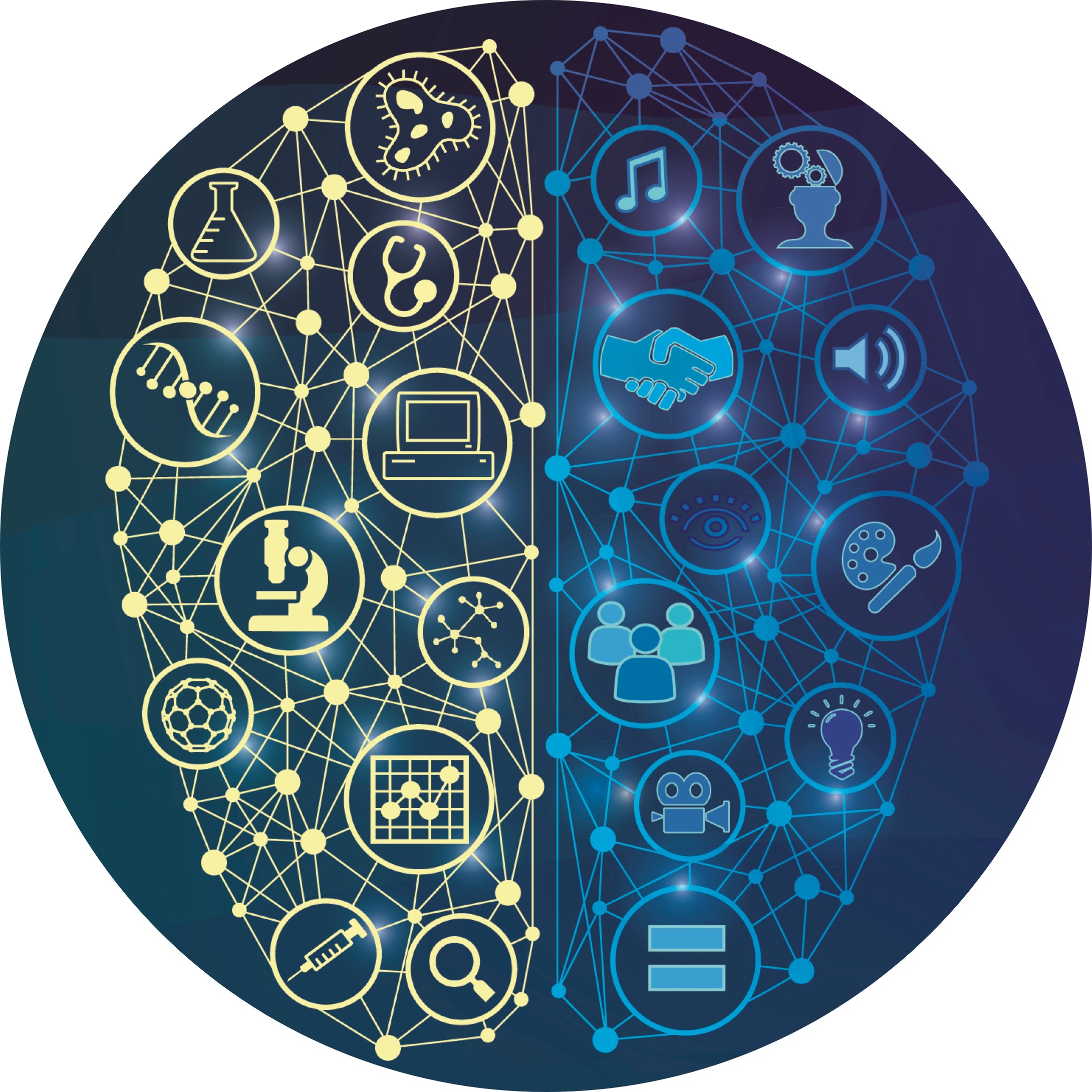