MFBM-MS07
From Machine Learning to Deep Learning Methods in Biology
Tuesday, June 15 at 09:30am (PDT)Tuesday, June 15 at 05:30pm (BST)Wednesday, June 16 01:30am (KST)
Organizers:
Erica Rutter (University of California, Merced, United States), Suzanne Sindi (University of California, Merced, United States)
Description:
As biological data becomes more detailed and ubiquitous, statistical and machine learning methods are needed to process and understand relationships in big data or to incorporate this data into existing mechanistic modeling frameworks. Here we present recent advances for machine learning and deep learning methodologies applied to a variety of biological processes, from single cell genomic analysis to population-wide disease spread. Methods of interest include biomedical image analysis via convolutional neural networks (CNNs), learning equations from data, and many more. The methods developed and discussed in this minisymposium span the range from purely statistical and machine learning models to hybridized mechanistic/machine learning models to data-driven mechanistic modeling.
Emilia Kozlowska
(Departement of Systems Biology and Engineering, Silesian University of Technology, Poland)"Application of mechanistic and machine learning modeling to predict long-term response to treatment for cancer patients"
Sara Ranjbar
(Mathematical NeuroOncology Lab, Precision Neurotherapeutics Program, Mayo Clinic, Arizona, United States)"MRI-based estimation of the abundance of immunohistochemistry markers in GBM brain using deep learning"
Joan Ponce
(UCLA, United States)"An integrated framework for building trustworthy data-driven epidemiological models: Application to the COVID-19 outbreak in New York City"
Emily Zhang
(North Carolina State University, USA)"Deep Learning and Regression Approaches to Forecasting Blood Glucose Levels for Type 1 Diabetes"
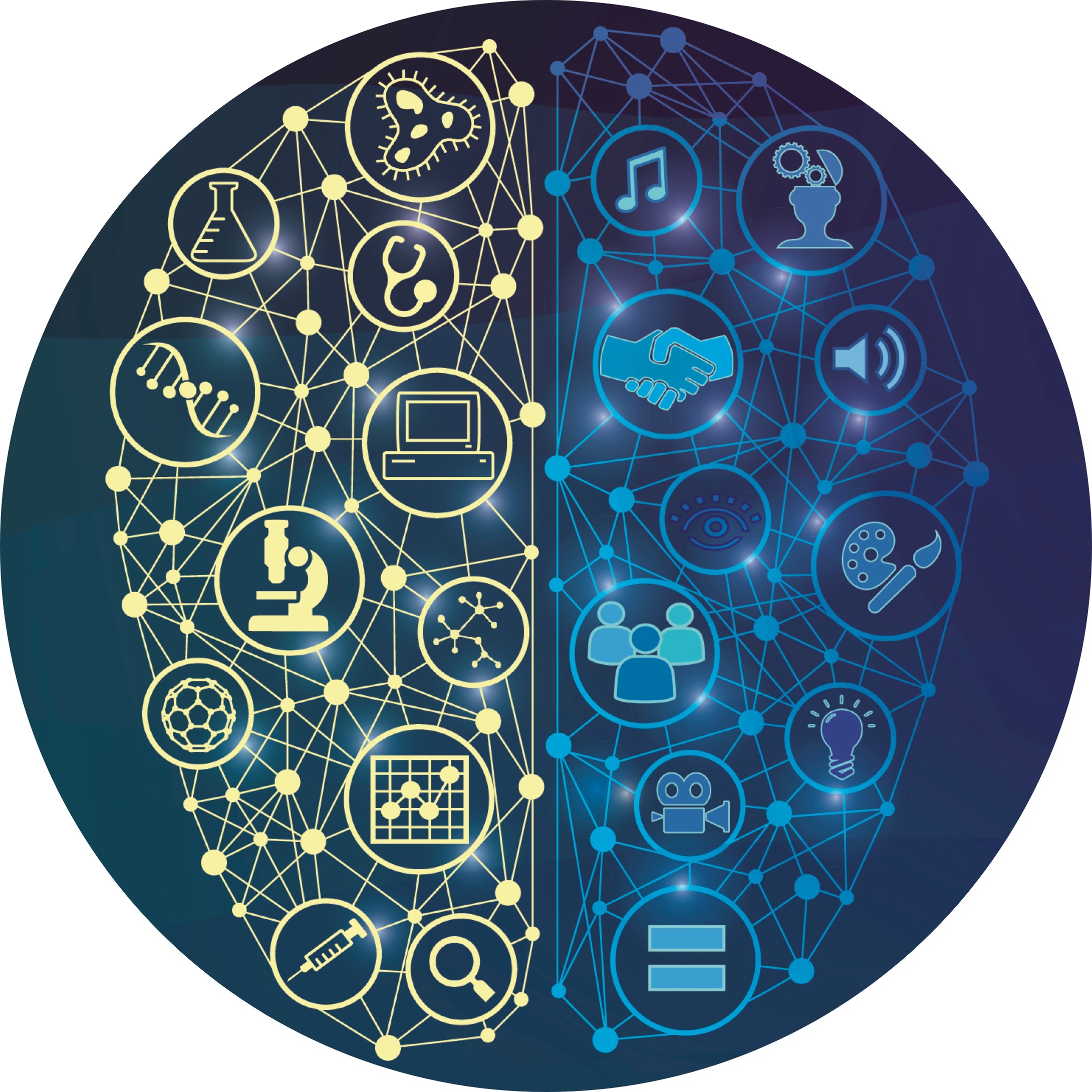