MFBM-MS08
From Machine Learning to Deep Learning Methods in Biology
Tuesday, June 15 at 11:30am (PDT)Tuesday, June 15 at 07:30pm (BST)Wednesday, June 16 03:30am (KST)
Organizers:
Erica Rutter (University of California, Merced, United States), Suzanne Sindi (University of California, Merced, United States)
Description:
As biological data becomes more detailed and ubiquitous, statistical and machine learning methods are needed to process and understand relationships in big data or to incorporate this data into existing mechanistic modeling frameworks. Here we present recent advances for machine learning and deep learning methodologies applied to a variety of biological processes, from single cell genomic analysis to population-wide disease spread. Methods of interest include biomedical image analysis via convolutional neural networks (CNNs), learning equations from data, and many more. The methods developed and discussed in this minisymposium span the range from purely statistical and machine learning models to hybridized mechanistic/machine learning models to data-driven mechanistic modeling.
Ali Heydari
((i) UC Merced Department of Applied Mathematics and (ii) Health Sciences Research Institute at UC Merced, USA)"Deep Generative Models for Realistic Single-Cell RNA-Seq Data Augmentation"
Mohammad Jafari
(Postdoctoral Scholar, Department of Applied Mathematics Jack Baskin School of Engineering University of California, Santa Cruz, USA)"Machine Learning-based Feedback Controller for Directing Stem Cell Membrane Potential"
Thomas de Mondesir
(Université Claude Bernard Lyon 1 & UC Merced, France)"Generating biological images to train deep-learning-based segmentation models"
Jordan Collignon
(University of California, Merced, USA)"A High-throughput Pipeline for Analyzing Experimental Images of Sectored Yeast Colonies"
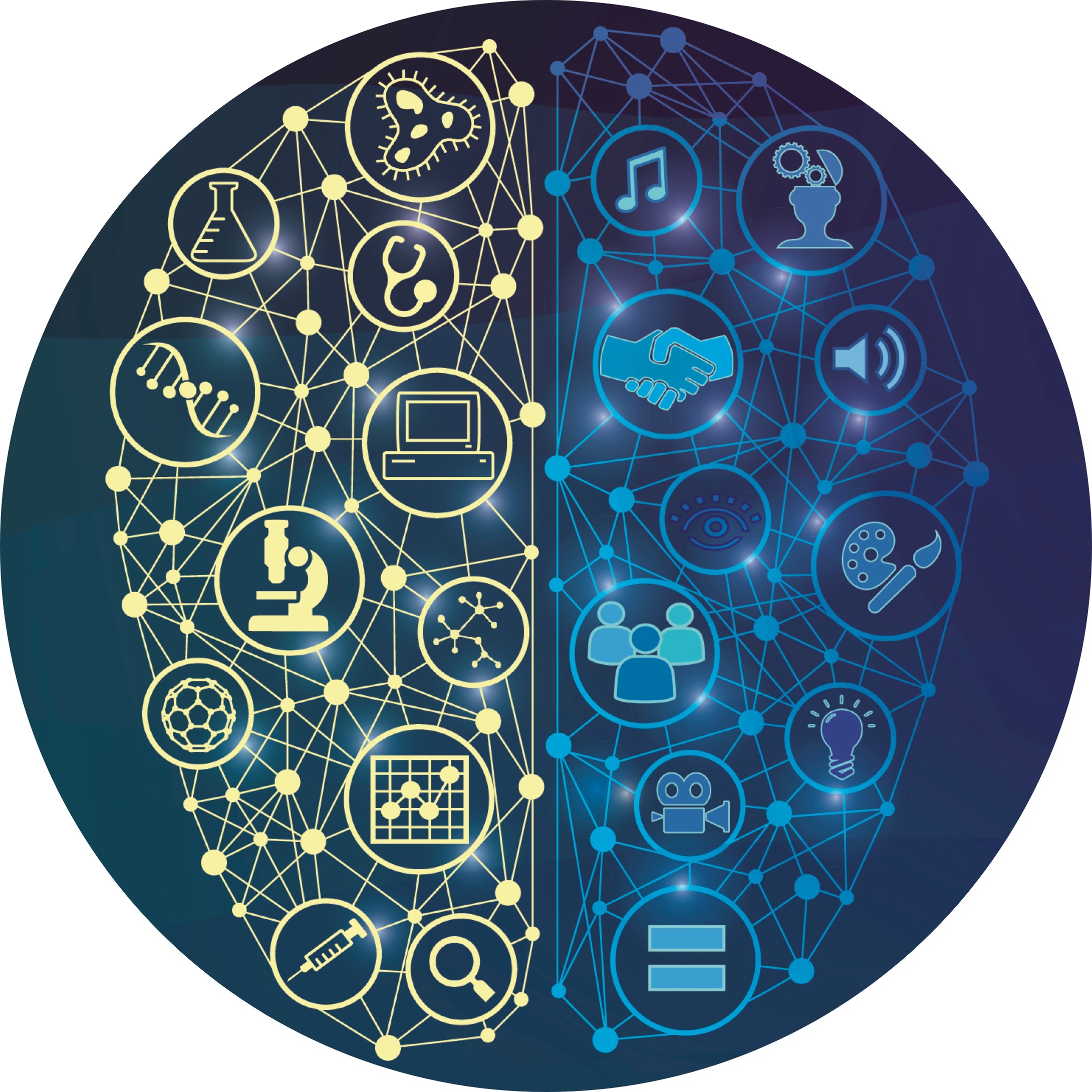