DDMB-MS16
Image Analysis and Machine Learning for Bio-Medical Applications
Wednesday, June 16 at 07:45pm (PDT)Thursday, June 17 at 03:45am (BST)Thursday, June 17 11:45am (KST)
Organizers:
Amit Roy-Chowdhury (University of California, Riverside), G. Venugopala Reddy (University of California, Riverside)
Description:
Image analysis is common in many bio-medical research applications. In spite of the prevalence of a number of relevant tools, a large part of the analysis is still manual and extremely tedious. This limits the amount of data that can be analyzed and prevents drawing statistically relevant conclusions. The reasons for the existing computational tools to not perform satisfactorily in many applications are often driven by poor image quality, and this calls for developing advanced methods that would be able to overcome these limitations. There has been tremendous progress in image analysis in the last decade, building upon machine learning methods. A pressing question is how do we translate these methods from the computer science fields into application in biomedical domains? This workshop will focus on such issues. Biomedical applications bring their own challenges that these advance computing approaches will need to address. In this workshop, we will focus on a few of these problems, e.g., how to use machine learning when there is limited labeled data, how do we combine physics-driven approaches with data-driven methods, and how do we combine human feedback effortlessly into the learning paradigm.
B. S. Manjunath
(University of California, Santa Barbara)"3D cell/nuclei segmentation and tracking using deep networks"
Michelle Digman
(University of California, Irvine)"Quantifying Spatio-temporal dynamics and Metabolic Alterations of protein upon DNA Damage"
Cory Braker Scott
(University of California, Irvine)"Morphological Analysis of Biological Images Using Spectral Graph Theory and Graph Neural Networks"
Kevin Rodriguez
(University of California, Riverside)"Interplay between layer specific chemical signals and mechanical properties maintain the structure and shape of the shoot apical meristem in Arabidopsis"
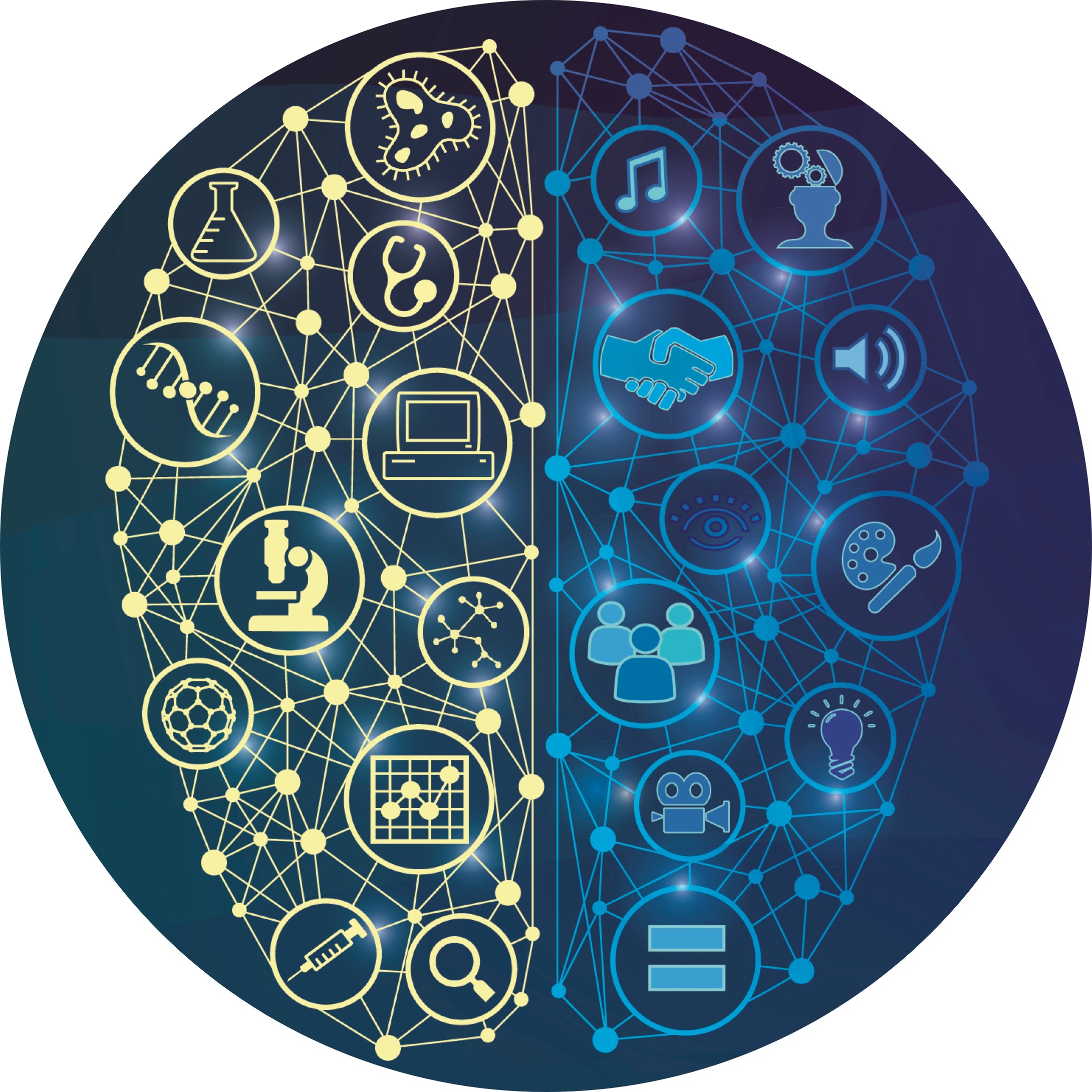