ONCO-MS06
Blackboard to Bedside: Showcase of Translational Modeling
Tuesday, June 15 at 04:15am (PDT)Tuesday, June 15 at 12:15pm (BST)Tuesday, June 15 08:15pm (KST)
Organizers:
Renee Brady-Nicholls (Moffitt Cancer Center, USA), Mohammad Zahid (Moffitt Cancer Center, USA), Stefano Pasetto (Moffitt Cancer Center, USA)
Description:
One of the most significant mathematical oncology capabilities lies in its ability to translate to the clinic and make patient-specific treatment recommendations that will ultimately prolong patient response and survival. This capability requires using clinical data as inputs to mathematical models to characterize individual patient responses and recommend clinically-relevant personalized treatment approaches and schedules. This common goal can be accomplished using various techniques. This mini-symposium will feature a varied selection of talks demonstrating how different approaches can support oncologists and patients in making clinical decisions. We will also highlight how this can improve the clinical trial design and how in silico modeling can investigate alternative therapy options.
Rene Bruno
(Genentech-Roche, France)"Tumor dynamic modeling and overall survival predictions to support decisions in oncology clinical trials"
Pamela Jackson
(Mayo Clinic, USA)"Instantiating an Imaging Digital Twin for a Brain Tumor Patient"
Elsa Hansen
(Penn State Huck Institutes of the Life Sciences, USA)"Maintenance therapy: A case study in trial design"
Sarah Brüningk
(ETH Zurich, Switzerland)"Intermittent radiotherapy as alternative treatment for recurrent high grade glioma: A modeling study based on longitudinal tumor measurements"
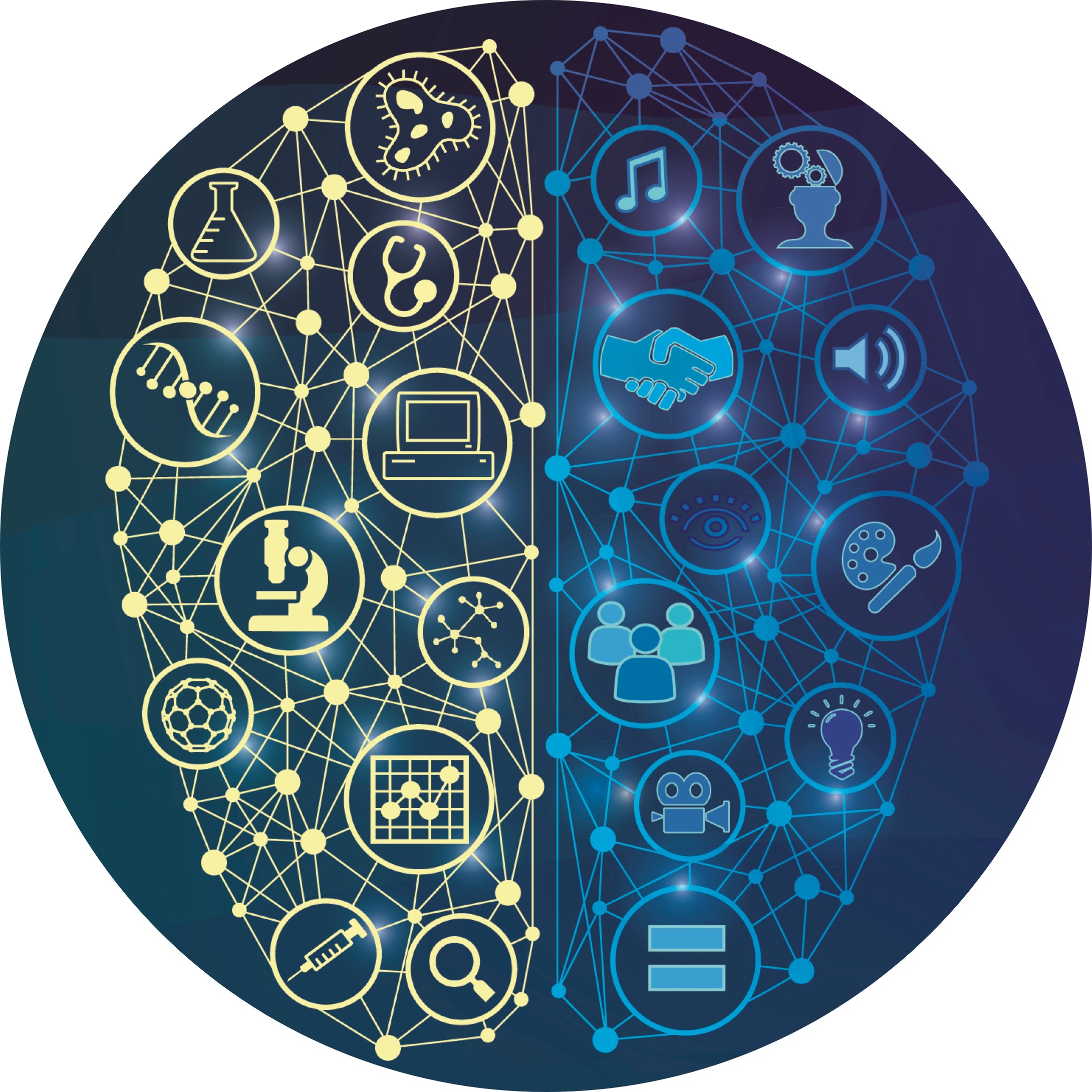