MMPB-MS06
How can mathematical modelling aid medical decision making?
Tuesday, June 15 at 04:15am (PDT)Tuesday, June 15 at 12:15pm (BST)Tuesday, June 15 08:15pm (KST)
Organizers:
Jasmina Panovska-Griffiths (University of Oxford), Eduard Campillo-Funollet (University of Sussex)
Description:
Mathematical and computational modelling can mimic real-life medical and biological environments to help us understand temporal and spatial behaviours and aid future predictions. The essence of modelling lies in designing sophisticated models that can help us replicate the biology and physiology we know, and allow us to make wise and reliable future decisions. In this mini-symposium we will showcase how mathematical modelling can be used to personalise radiotherapy, control the spread of the current pandemic, aid the process of deep tissue healing without scarring and improve non-invasive diagnosis of brain gliomas. Our speakers will take time to put the modelling in the context of real-life, discuss the theoretical framework of their models and outline the policy decision applications. The key of the modelling we will showcase is that it is currently being used by policy decision makers and for diagnostic and prognostic purposes. Therefore, the overarching aim of this mini-symposium is not only to showcase the specific models and the mathematics behind them, but also to discuss that creating a model requires far more than just raw data and technical skills; it also requires a close collaboration between mathematicians/computational scientists with clinical researchers and policy decision makers. Specifically, a good model requires robust theoretical framework, but also flexible multidisciplinary collaboration. The speakers will show how this is done in their research fields. Modern modelling approaches are a powerful tool for decision making, especially in this era of ”big data” and in the face of uncertainty. Therefore, in the course of this symposium we will showcase a range of topics revolving around medical and health applications of modern mathematical modelling, including cell biology, epidemiology and diagnostics, with the common denominator aiming to improve the medical decision making process and public health.
Elizabeth Ford
(Brighton and Sussex Medical School)"Can modelling of primary care patient records enable detection of dementia earlier than the treating physician?"
Robin Thompson
(University of Warwick)"Can modelling be used to predict whether or not the novel coronavirus will spread in the UK?"
Fred Vermolen
(Delft University of Technology)"Can modelling aid the process of deep tissue healing without scarring?"
Jasmina Panovska-Griffiths
(University of Oxford)"Can combining modelling and brain radiomics non- invasively stratify brain gliomas?"
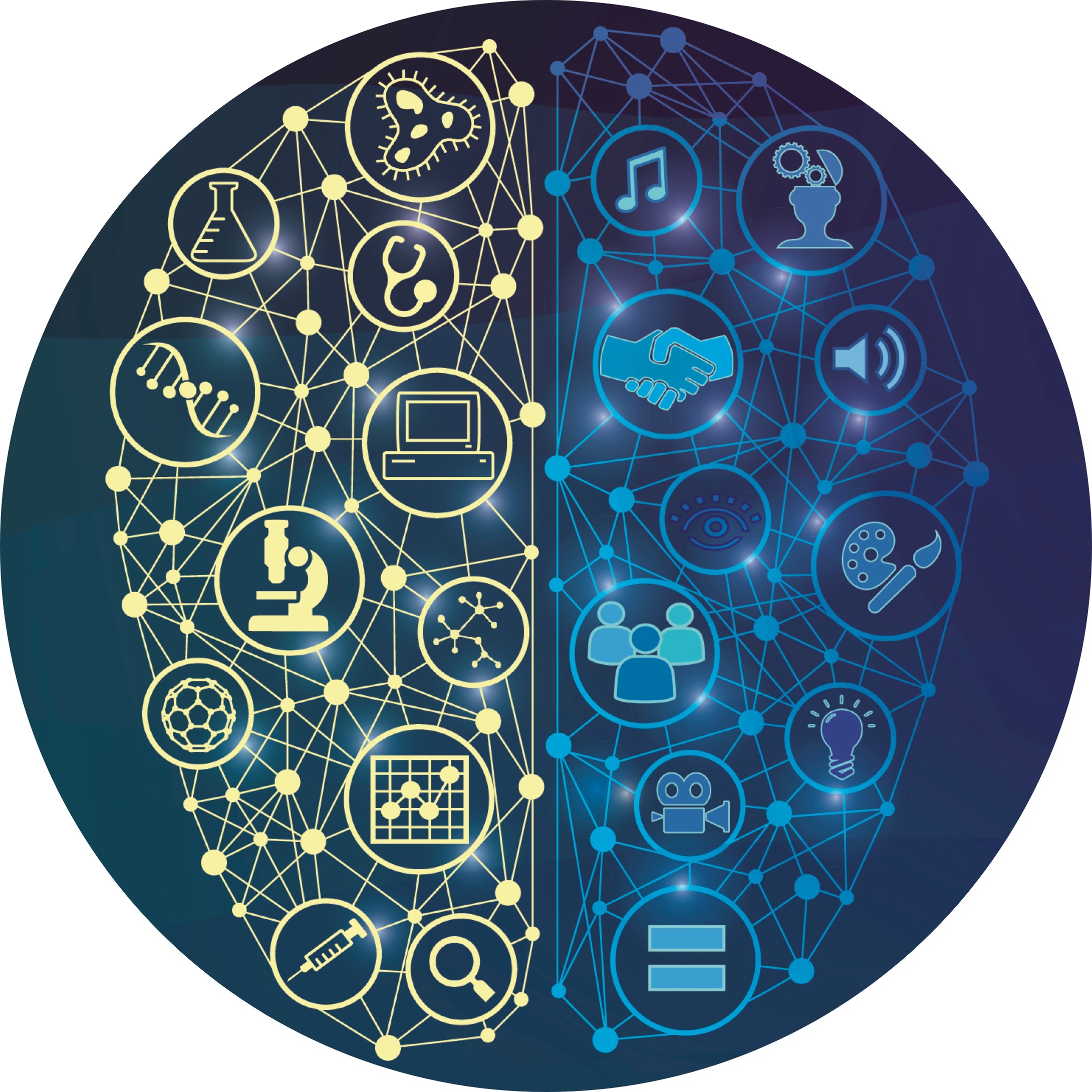