SMB2021
Lee A. Segel Prize for Best Paper & Student Paper
Introduced by: Ruth BakerTuesday, June 15 at 01:15am (PDT)Tuesday, June 15 at 09:15am (BST)Tuesday, June 15 05:15pm (KST)
Plenary-03 : Lee A. Segel Prize for Best Paper & Student Paper
Presentations from Paper Prize Winners
Best paper:
Link to best paperPaper: "A Multi-stage Representation of Cell Proliferation as a Markov Process" by Christian A. Yates*, Matthew J. Ford, Richard L. Mort
Adventures in developmental biology.
In this talk I will give a number of short vignettes of work that has been undertaken in my group over the last 15 years. Mathematically, the theme that underlies our work is the importance of randomness to biological systems. I will explore a number of systems for which randomness plays a critical role. Models of these systems which ignore this important feature do a poor job of replicating the known biology, which in turn limits their predictive power. The underlying biological theme of the majority our work is development, but the tools and techniques we have build can be applied to multiple biological systems and indeed further afield. Topics will be drawn from, locust migration, zebrafsh pigment pattern formation, mammalian cell migratory defects, appropriate cell cycle modelling and more. I won't delve to deeply into anyone area, but am happy to take question or to expand upon of the areas I touch on.
Best student paper:
Link to best student paperPaper: "A Bayesian Sequential Learning Framework to Parameterise Continuum Models of Melanoma Invasion into Human Skin" by Alexander P. Browning*, Parvathi Haridas, Matthew J. Simpson
Tackling biological and model complexity through a sequence of experiments
Biological and model complexity is a major challenge in mathematical biology. While more complex models capture the finer details in biological processes, they typically suffer from practical non-identifiability when calibrated to typical experimental measurements. We demonstrate this for a simple model of melanoma invasion into human skin, where it is challenging to extract detailed biological measurements from experimental images. We demonstrate an approach to alleviate this problem through a Bayesian sequential learning framework, where a sequence of mathematical and experimental models are applied in tandem to gradually learn about key components of the process and calibrate the full mathematical model. Whereas parameter estimates from a single melanoma invasion experiment are meaningless, those from our sequential learning approach are well-defined and describe cell behaviour throughout the sequence of experiments we consider.
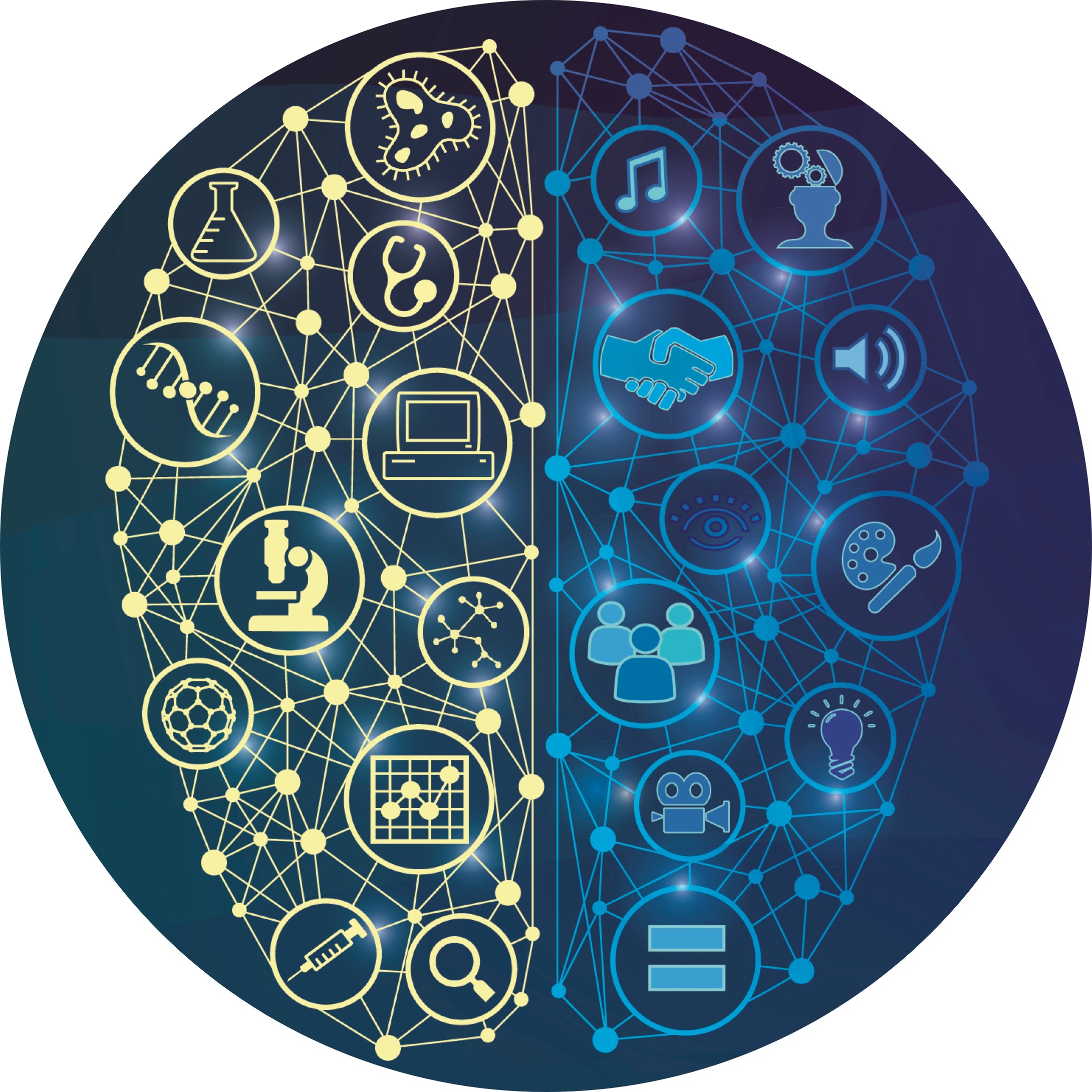