ONCO-
Applications and challenges of using quantitative imaging data for biologically-based mathematical oncology
Organizers:
David A Hormuth II, Guillermo Lorenzo
Description:
Quantitative imaging is being increasingly used to improve cancer diagnosis, monitoring, and treatment planning. In particular, the advent of multiparametric imaging has provided a noninvasive means to obtain a wealth of time- and spatially-resolved quantitative data about tumor morphology, architecture, vascularity, and dynamics. These unique data types collected over time can also be leveraged to predict the evolution of an individual patient’s tumor. Indeed, in recent years there has been extensive use of medical imaging data to inform, initialize, and calibrate biophysical models of tumor growth and treatment response [1,2]. These models aim to characterize patient or disease specific growth properties (e.g., diffusion, invasion, and proliferation rates) and patient or disease specific response to therapy (e.g., radiotherapy, chemotherapy, immunotherapy). There are even emerging methods to use model-based forecasts of response to enable physicians to optimally design the best managing strategy for each patient ́s tumor. Quantitative imaging has also been used to provide in vivo validation of modeling hypotheses, hence extending our understanding of tumor dynamics. In this mini- symposium we will discuss (1) translating pre-clinical models to clinical disease (2) modeling response to radiotherapy from in vitro to in vivo settings, (3) assessing the clinical utility of patient-specific response metrics, and (4) constructing imaging biomarkers grounded on mathematical models.
David A. Hormuth, II
The University of Texas at Austin, Austin, Texas USA"Translating image driven models of response to radiation therapy from the pre-clinical to clinical setting"
Sarah Brüningk
Institute of Molecular Systems Biology, ETH Zurich, Zurich Switzerland"Intermittent radiotherapy as alternative treatment for recurrent high grade gliomas: A modelling study based on longitudinal tumour measurements"
Andrea Hawkins-Daarud
Precision Neurotherapeutics Innovation Program, Mayo Clinic, USA"Assessing clinical utility of a model based patient-specific response metric for glioblastoma incorporating uncertainty quantification from image acquisition and segmentation"
Victor M. Perez-Garcia
Universidad de Castilla – La Mancha, Spain"If you have mathematical models then you have imaging biomarkers: Applications to gliomas, lung cancer, breast cancer and head & neck cancer"
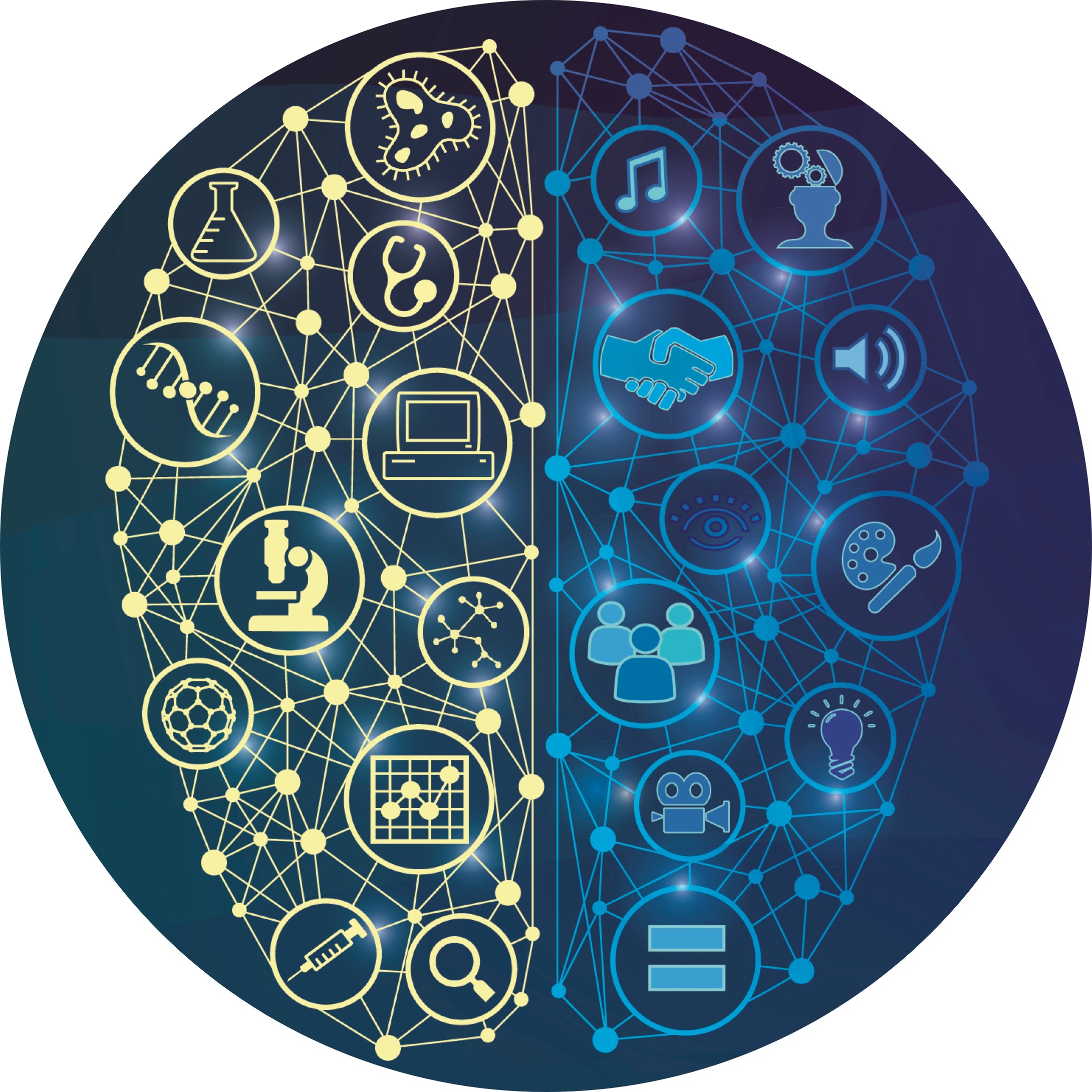