Share this
Pirmin Schlicke
Chair for Mathematical Modeling at the Center of Mathematics, Technical University of Munich, Germany"How Mathematical Modeling Could Contribute to the Quantification of Metastatic Tumor Burden Under Therapy"
Maximilian Strobl
Department of Integrated Mathematical Oncology, H. Lee Moffitt Cancer Center Tampa, USA"Personalising adaptive cancer therapy in theory and in practice: the role of resistance costs and cellular competition"
Johannes Reiter
Stanford University School of Medicine, Stanford, USA"A mathematical model of ctDNA shedding predicts tumor detection size"
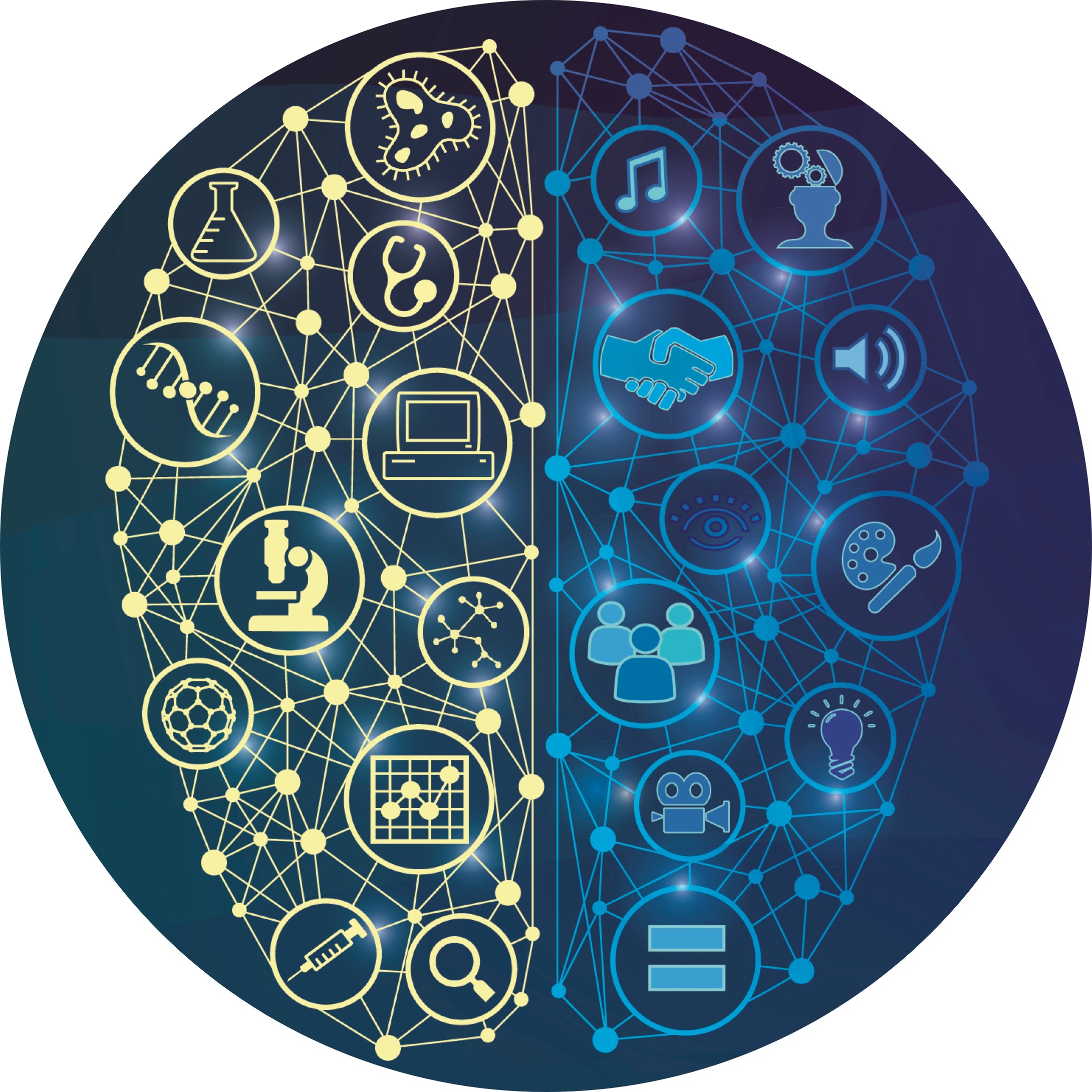