ONCO-MS14
Integrating quantitative imaging and mechanistic modeling to characterize tumor growth and therapeutic response
Wednesday, June 16 at 11:30am (PDT)Wednesday, June 16 at 07:30pm (BST)Thursday, June 17 03:30am (KST)
Organizers:
Guillermo Lorenzo (University of Pavia, Italy), David Hormuth (The University of Texas at Austin, US), Angela Jarrett (The University of Texas at Austin, US), Thomas Yankeelov (The University of Texas at Austin, US)
Description:
The overall goal of this symposium is to present and discuss recent developments in (1) the integration of imaging data in mechanistic models to investigate cancer development and therapeutic response both in vitro and in vivo, (2) translating pre-clinical image-based models to clinical disease, and (3) assessing image-based and model-inspired biomarkers and response metrics to improve clinical decision-making and to advance (pre)clinical research. Cancers are highly heterogeneous diseases supported by diverse biological mechanisms occurring, interacting, and evolving at multiple spatial and temporal scales. Quantitative imaging provides a noninvasive means to characterize this heterogeneous, multiscale nature by providing a wealth of temporally and spatially resolved data about morphology, architecture, vascularity, growth dynamics, and response to therapy. Hence, quantitative imaging is being increasingly used to improve cancer diagnosis, monitoring, and treatment planning. Additionally, these imaging technologies are accelerating in vitro and in vivo research on the biological mechanisms underlying the development and therapeutic response of tumors. Quantitative imaging data can be further exploited to constrain biophysical models of tumor growth and treatment response both in preclinical and clinical settings. These models can then be leveraged to test hypotheses, produce individualized tumor forecasts to guide clinical decision-making, and, ultimately, to design optimized therapies.
Andrea Gardner
(The University of Texas at Austin, US)"Quantification of interactions between epithelial-like and mesenchymal-like subpopulations in a triple-negative breast cancer cell line ecosystem"
Haley Bowers
(Wake Forest School of Medicine, US)"Image Data-Driven Biophysical Mathematical Model Based Characterization of Multicellular Tumor Spheroids"
Anum Kazerouni
(University of Washington, US)"Characterizing tumor heterogeneity using quantitative MRI habitats in breast cancer in vivo"
David Hormuth
(The University of Texas at Austin, US)"Image-driven modeling of radiation therapy response in gliomas"
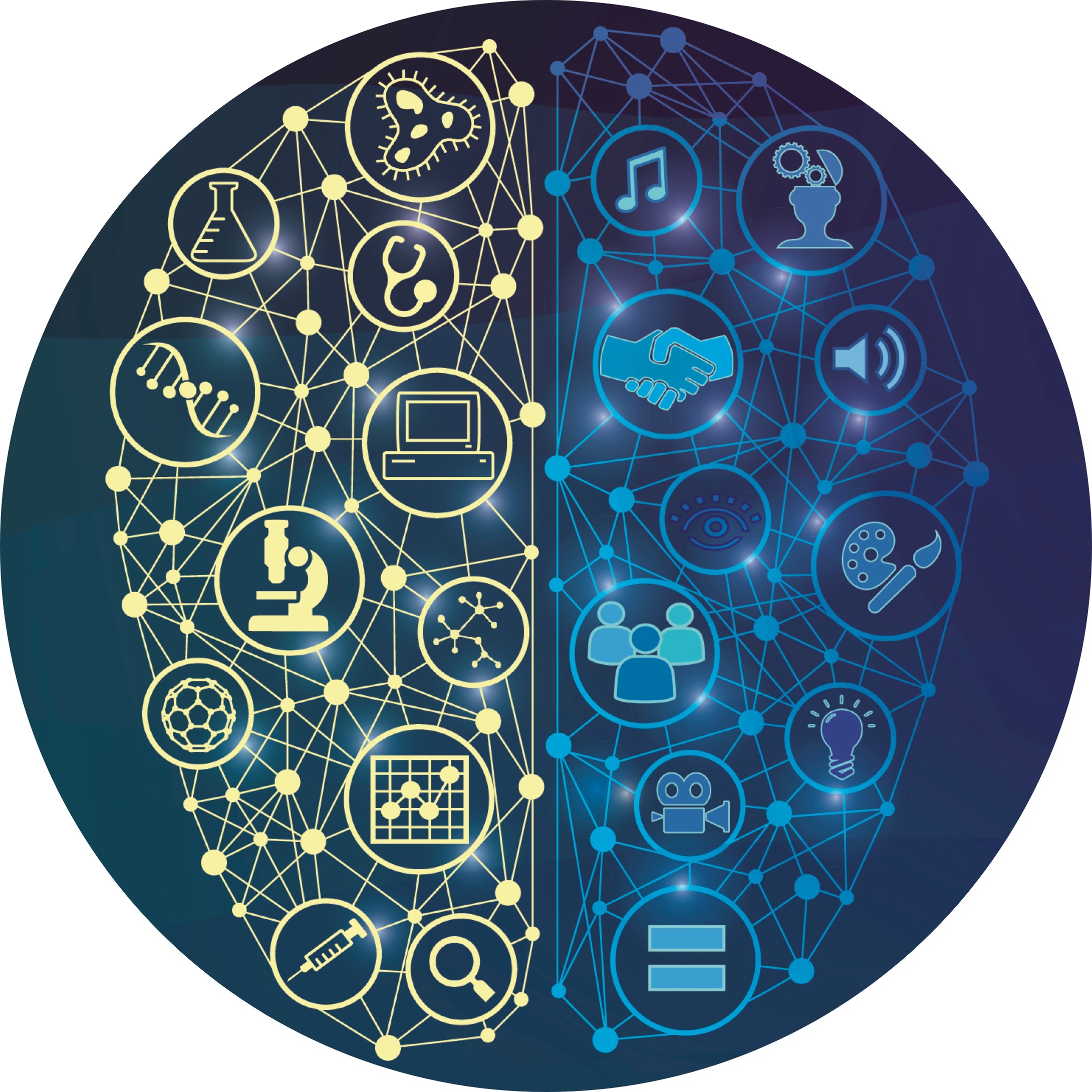