ONCO-CT07
ONCO Subgroup Contributed Talks
Wednesday, June 16 at 02:15pm (PDT)Wednesday, June 16 at 10:15pm (BST)Thursday, June 17 06:15am (KST)
Share this
Pirmin Schlicke
Technical University of Munich, Germany"Bringing math into medical clinics: a model framework quantifying treatment outcomes in metastatic cancer"
Daniel Glazar
Moffitt Cancer Center"Predicting Advanced Head and Neck Cancer Patients with High Risk of Early Treatment Failure"
Maximilian Strobl
University of Oxford & Moffitt Cancer Center"Using eco-evolutionary modelling to improve the management of PARPi resistance in ovarian cancer maintenance therapy"
Ryan Murphy
Queensland University of Technology"Looking beneath the surface of tumour spheroids: insights from mathematical models parameterised to experimental data"
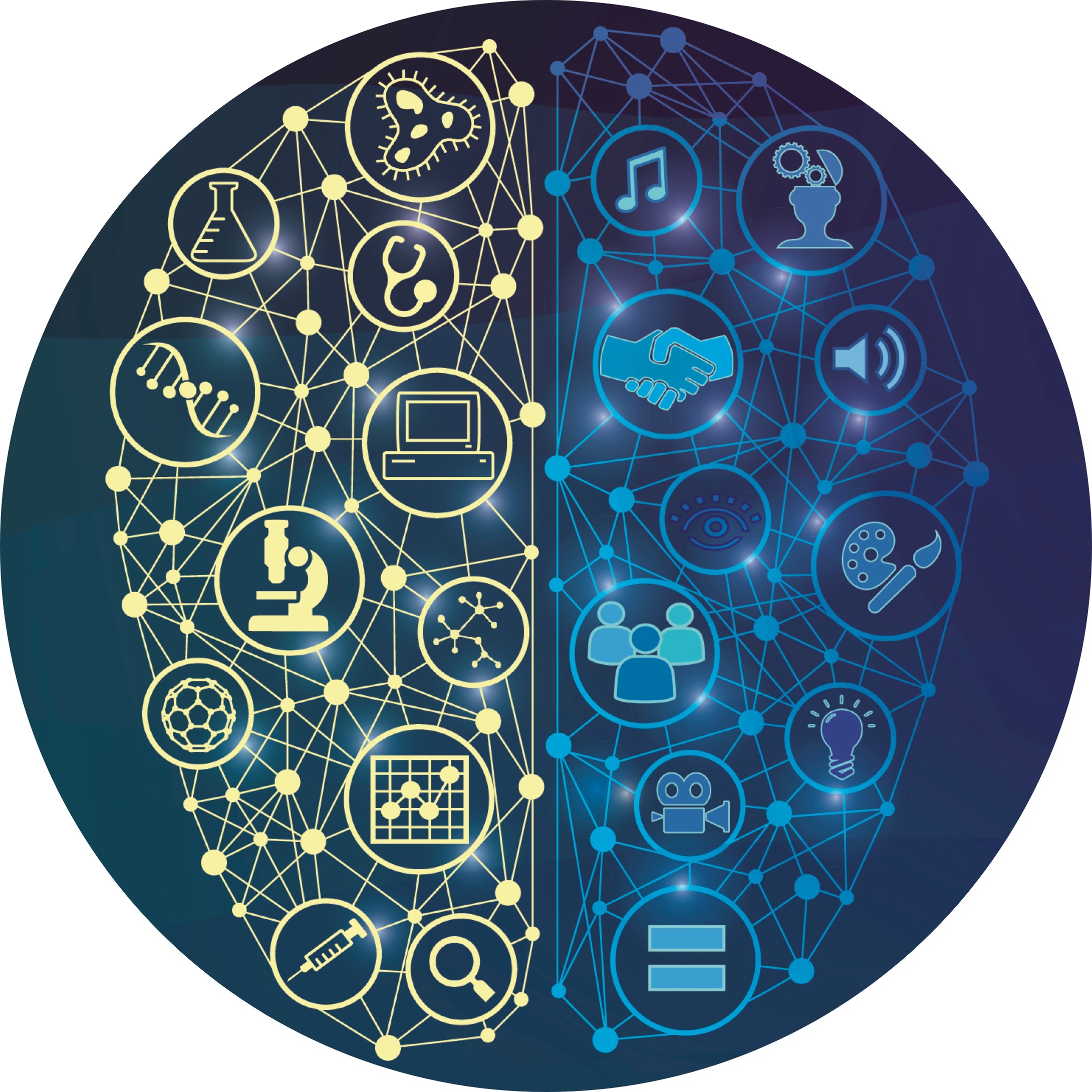