ONCO-CT05
ONCO Subgroup Contributed Talks
Tuesday, June 15 at 10:30pm (PDT)Wednesday, June 16 at 06:30am (BST)Wednesday, June 16 02:30pm (KST)
Share this
Maalavika Pillai
Indian Institute of Science, Bangalore"Systems-level analysis of phenotypic plasticity and heterogeneity in melanoma"
Jill Gallaher
Moffitt Cancer Center"Using adaptive therapy to characterize collective and individual characteristics of metastases"
Joshua Bull
Wolfson Centre for Mathematical Biology, University of Oxford"Novel spatial statistics describe phenotype transitions in an agent-based model of tumour associated macrophages"
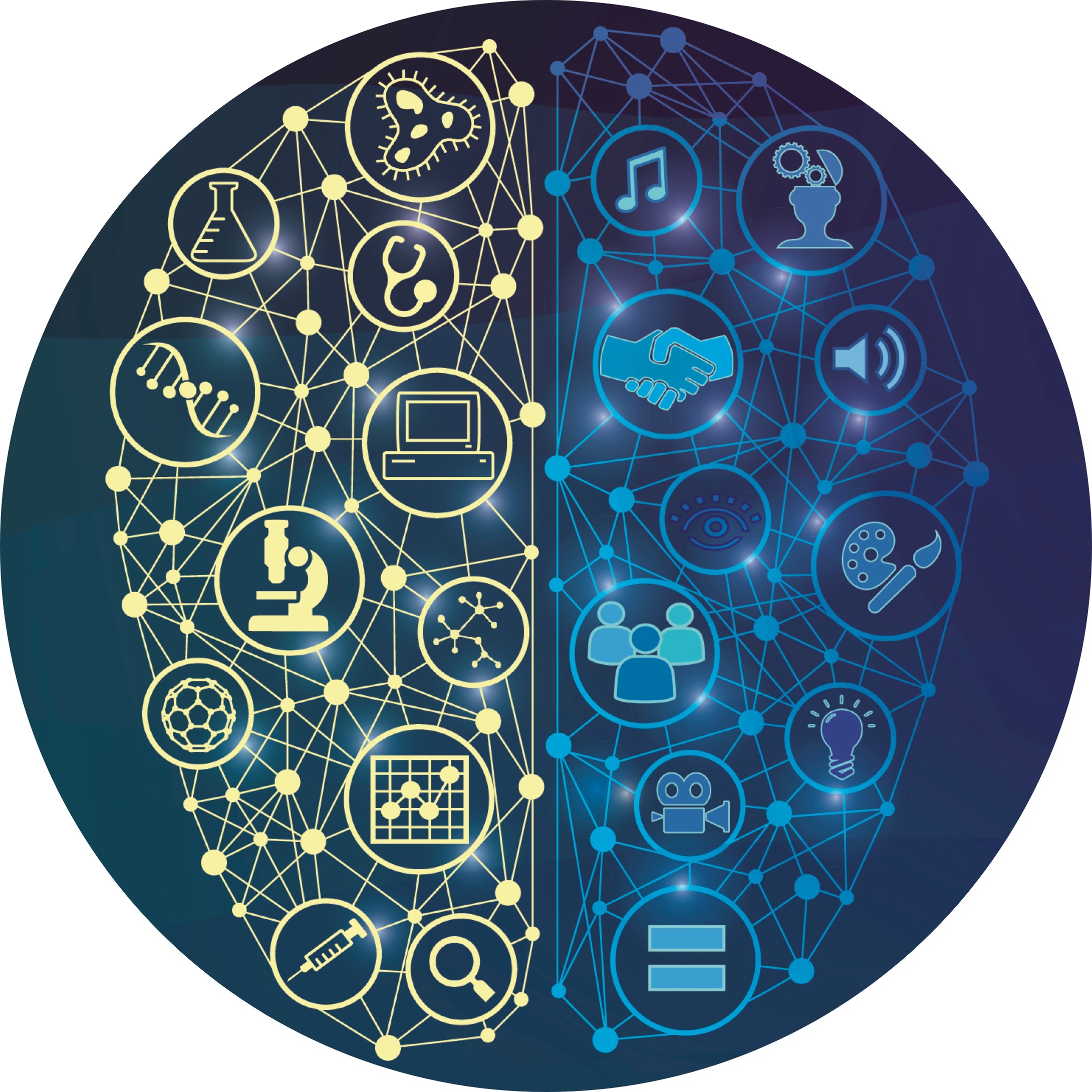