NEUR-MS05
Recent advances in mathematical neuroscience: cortically inspired models for vision and synaptic plasticity
Tuesday, June 15 at 02:15am (PDT)Tuesday, June 15 at 10:15am (BST)Tuesday, June 15 06:15pm (KST)
Share this
Organizers:
Luca Calatroni (Laboratoire I3S, CNRS, UCA & Inria Sophia Antipolis Méditerranée, France), Mathieu Desroches (MathNeuro Project-Team, Inria Sophia Antipolis Méditerranée & Université Côté d’Azur, France), Valentina Franceschi (Dipartimento di Matematica, Università degli Studidi Padova, Italy), Dario Prandi (Université Paris-Saclay, CNRS, CentraleSupélec, L2S, France)
Description:
The purpose of this symposium is to gather together experts working in the field of mathematical neuroscience, with a focus on those working on cortical inspired models for vision and synaptic plasticity. In particular the speakers will present recent results on variational and differential approaches to the understanding of the primary visual cortex as well as more recent models based on neural networks and predictive coding.
Laurent Perrinet
(INT, CNRS - Aix-Marseille Université, France)"Pooling in a predictive model of V1 explains functional and structural diversity across species"
Rufin Van Rullen
(CerCo, CNRS and ANITI, Universite de Toulouse, France)"Deep predictive coding for more robust and human-like vision"
Yuri Elias Rodrigues
(INRIA/IPMC/Université Côte d'Azur, France)"Modelling the experimental heterogeneity of synaptic plasticity"
Halgurd Taher
(Inria Sophia Antipolis-Méditerranée Research Centre, France)"Bursting in a next generation neural mass model with synaptic dynamics: a slow-fast approach"
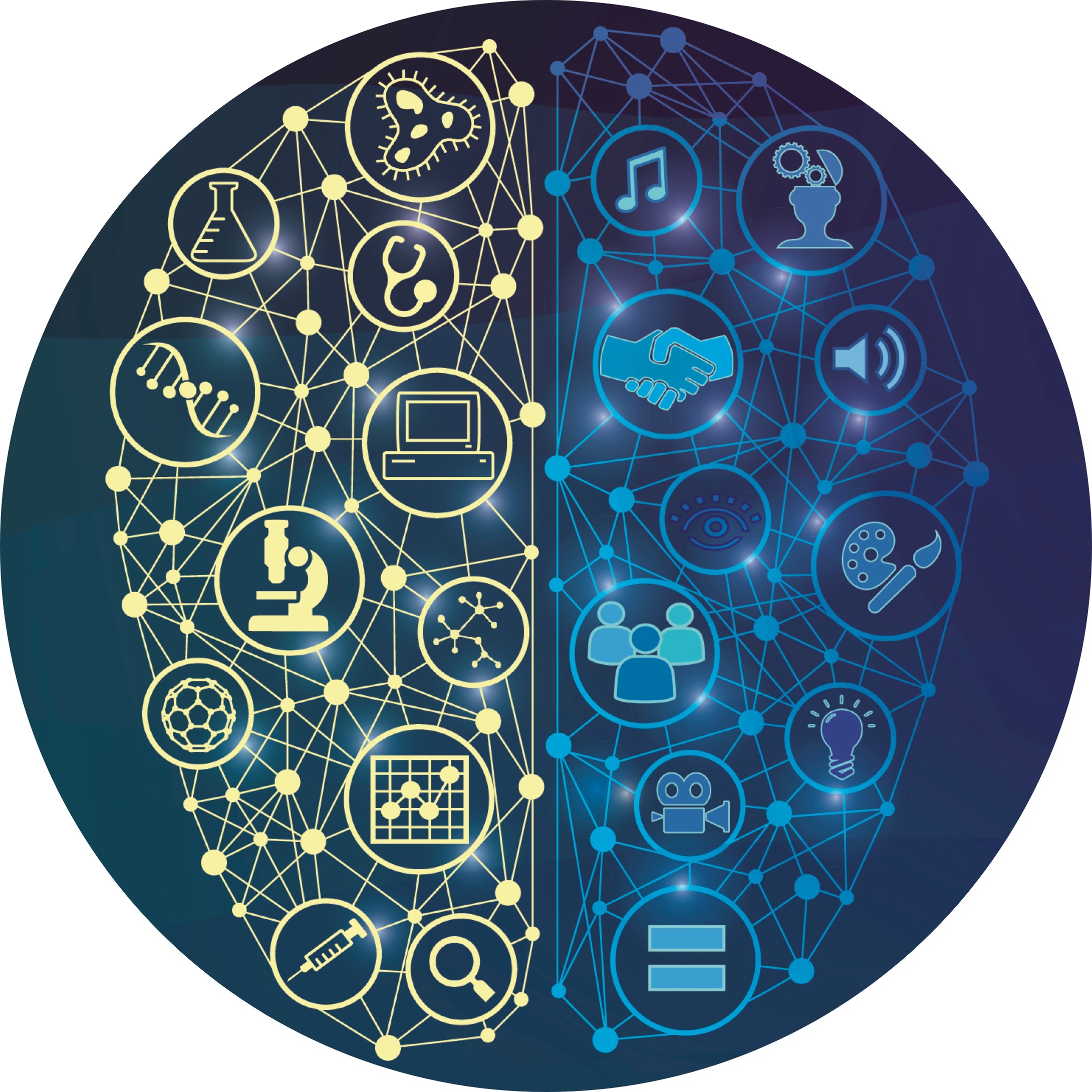