MFBM-MS14
Data-driven methods for biological modeling in industry
Wednesday, June 16 at 11:30am (PDT)Wednesday, June 16 at 07:30pm (BST)Thursday, June 17 03:30am (KST)
Organizers:
Kevin Flores (North Carolina State University, USA)
Description:
This minisymposium highlights recent advances in data-driven mathematical modeling for biology in industry, including parameter estimation, uncertainty quantification, machine learning, and image analysis. In particular, the emphasis is on the development of methods for overcoming practical challenges encountered with real-world data from industrial applications, such as high levels of observation error, model bias, and intra- as well as inter-individual or experimental heterogeneity. Topics include optimization of clinical dose regimens, optimal sample collection, forecasting, hypothesis testing and/or model selection, and the integration of heterogeneous sources of data from multiple scales and data acquisition platforms.
Richard Allen
(Quantitative Systems Pharmacology, Early Clinical Development, Pfizer Worldwide Research Development and Medical, USA)"Analyzing and Predicting Clinical Trial Data with Systems Modeling"
Florencio Serrano Castillo
(Clinical Pharmacology, Modelling and Simulations, Amgen Inc., USA)"Dosing guidance optimization, leveraging real world heterogeneity to forecast clinical biomarker response"
Zackary Kenz
(DILIsym Services, a Simulations Plus Company, USA)"Quantitative Systems Pharmacology Modeling of Fibrotic Diseases"
Anna Neely
(TigerRisk Partners, USA)"Estimating the growing risk of severe thunderstorms"
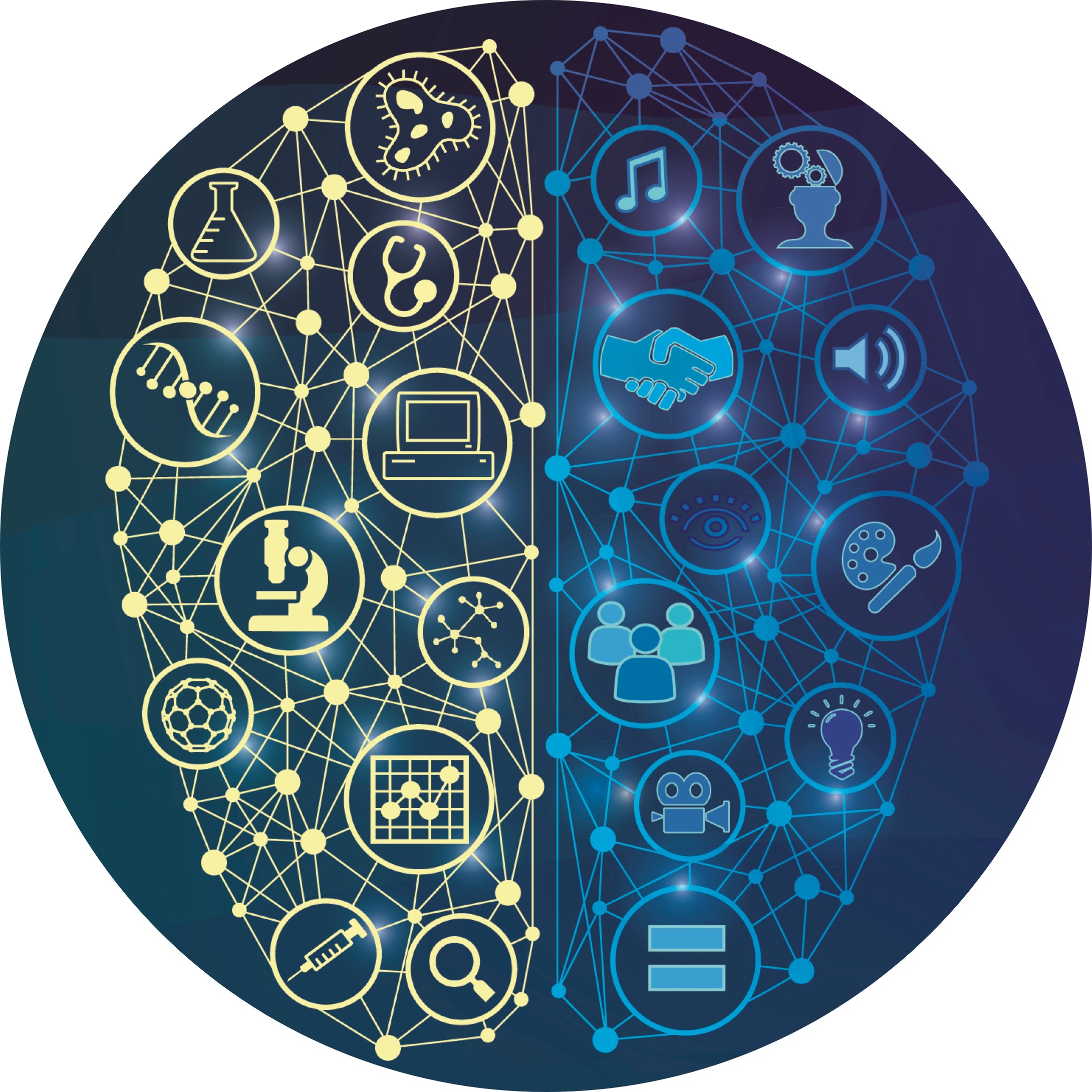