CDEV-MS14
Combining modeling and inference in cell biology
Wednesday, June 16 at 11:30am (PDT)Wednesday, June 16 at 07:30pm (BST)Thursday, June 17 03:30am (KST)
Organizers:
Maria-Veronica Ciocanel (Duke University, United States), John Nardini (North Carolina State University, United States)
Description:
In many cell and developmental processes, both modeling and data analytic approaches are necessary in order to generate useful modeling predictions to guide the design of further experiments for both validating and improving biological insight. There is an increased understanding that the application of machine learning methods can also be used to enhance common data-driven modeling techniques, including parameter and equation inference, classification, and sensitivity analysis. The speakers in this session will discuss how continuous differential equation models, individual-based stochastic models, and methods from machine learning can be used to address questions related to mitosis, intracellular transport, cell migration, and tissue development. The speakers will highlight current research progress and challenges associated with combining modeling and inference approaches in cell and developmental biology.
Keisha Cook
(Tulane University, United States)"Single Particle Tracking with applications to lysosome transport"
Christopher Miles
(New York University, United States)"Stochastic organization of the mitotic spindle from spatiotemporal trajectories"
Ruth Baker
(University of Oxford, United Kingdom)"Quantifying the impact of electric fields on single-cell motility"
Carter Jameson
(North Carolina State University, United States)"Parameterizing agent based models of collective cell migration using topological information"
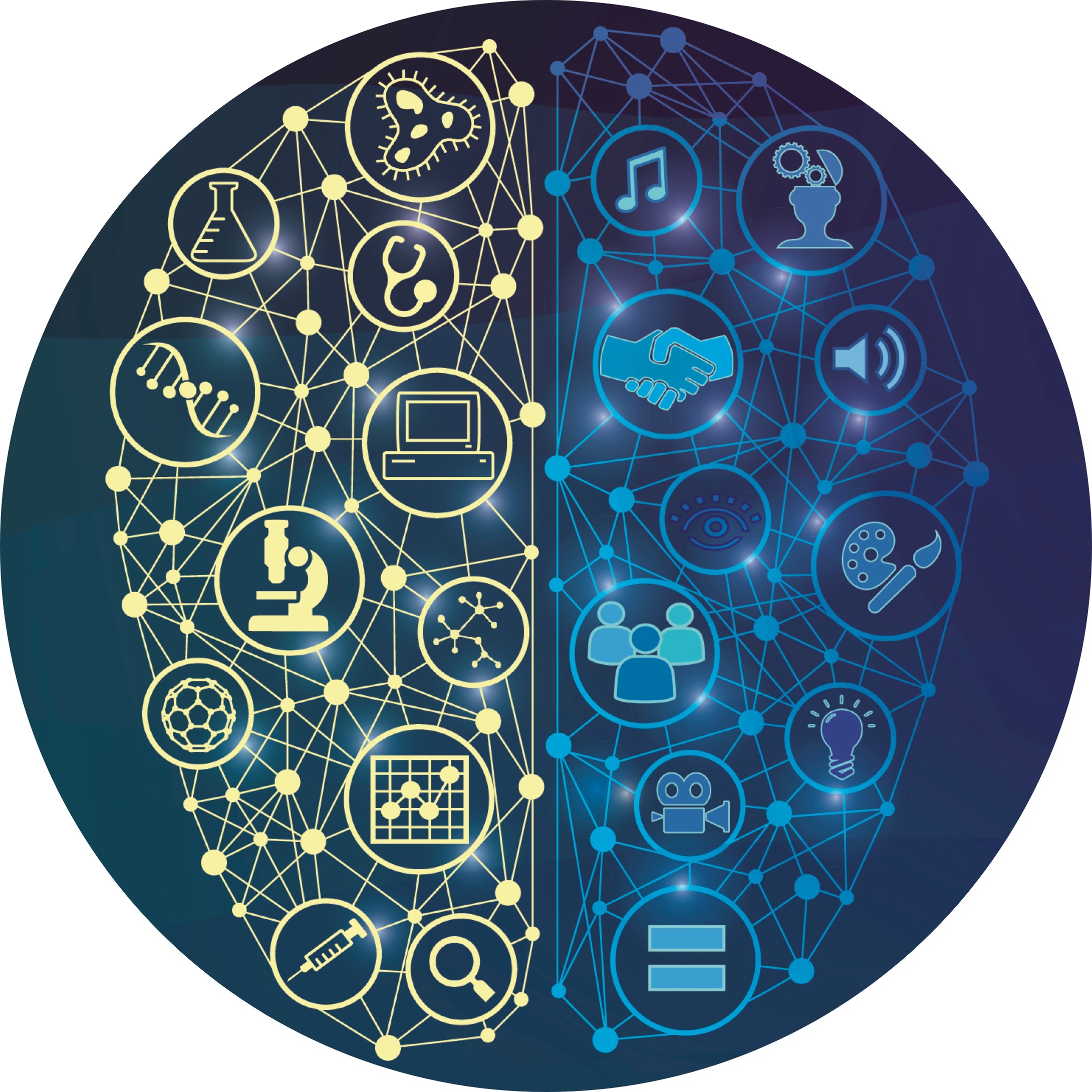