CBBS-MS09
Modeling and data analysis of dynamics from molecules, cells to populations
Tuesday, June 15 at 05:45pm (PDT)Wednesday, June 16 at 01:45am (BST)Wednesday, June 16 09:45am (KST)
Share this
Organizers:
Lei Zhang (Peking University, China)
Description:
The systems biology approach integrating heterogeneous biological data in quantitative mathematical models has promised to facilitate the comprehensive understanding of complex biological systems. This A3 (China-Japan-Korea) minisymposium is to bring together asian mathematicians working in the field of mathematical modeling and data analysis of dynamic phenomena at all kinds of levels on molecules, cells to populations.
Hao Ge
(Peking University, China)"The Nonequilibrium Mechanism of Noise-Enhanced Drug Synergy in HIV Latency Reactivation"
Yusuke Imoto
(Kyoto University, Japan)"Topological Trajectory Inference for Single-cell RNA Sequencing Data"
Suoqin Jin
(University of California Irvine, U.S.)"Understanding the role of cell-cell communication in cell fate decisions from single-cell data"
Dae Wook Kim
(Korea Advanced Institute of Science and Technology, Korea)"Moment-based inference of cell-to-cell variability in signal transduction time"
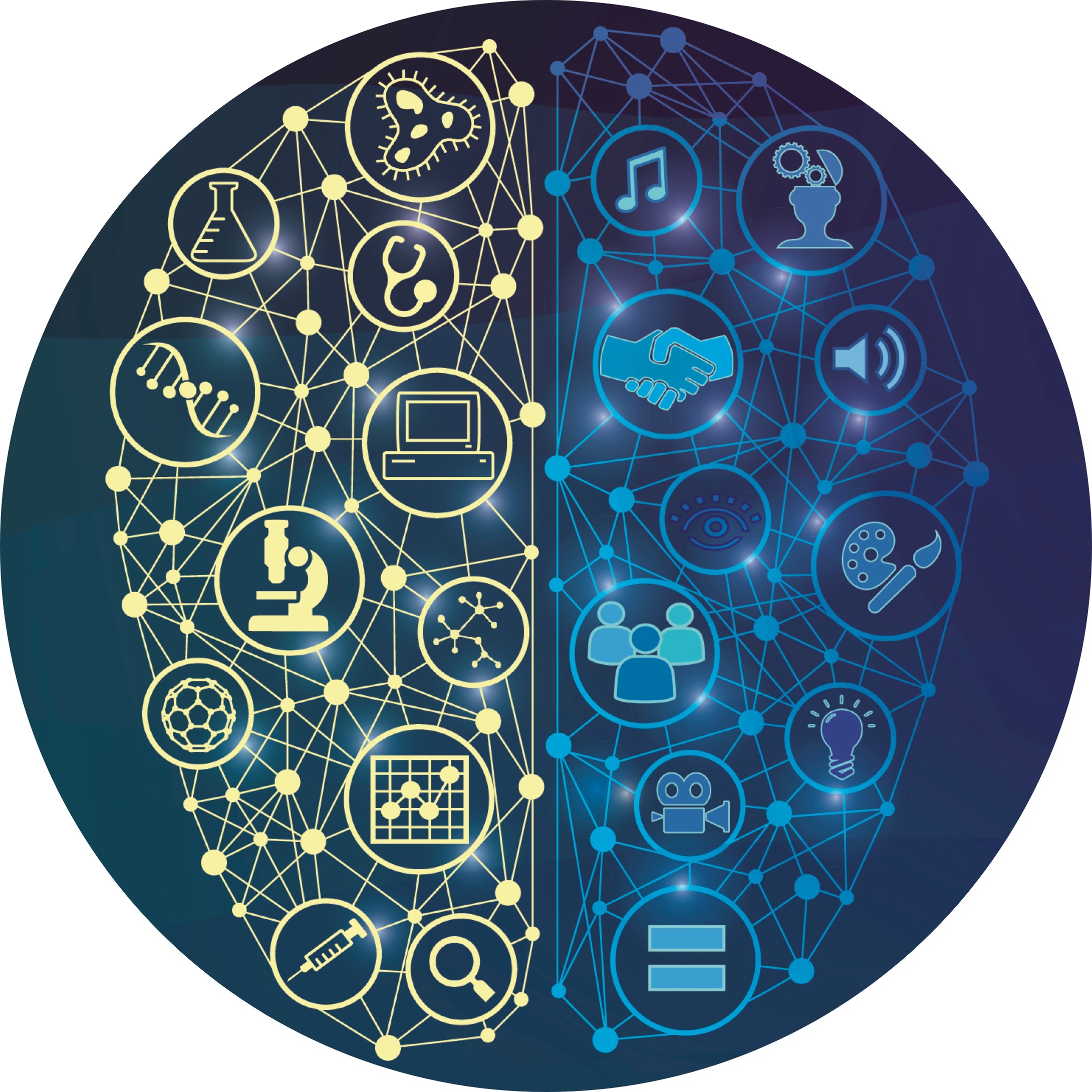