ONCO-MS07
Modeling translational oncology
Tuesday, June 15 at 09:30am (PDT)Tuesday, June 15 at 05:30pm (BST)Wednesday, June 16 01:30am (KST)
Share this
Organizers:
Russell Rockne (Beckman Research Institute, City of Hope National Medical Center, USA), Andrea Bild (Beckman Research Institute, City of Hope National Medical Center, USA)
Description:
This session focuses on application of mathematical models in cancer treatment and tumor biology. Integrated clinical, biological, and mathematical approaches will be discussed as they apply to tumor models. Results from these models will include optimization of treatment strategies directed at individual tumor features, quantification of evolving subclonal populations linked to therapy resistance, and prediction of treatment response to immunotherapy. Speakers from both academia and pharmaceutical research centers will together discuss emergent opportunities for growth in this field.
Jessica Leete
(Pfizer Inc, Cambridge MA, USA)"Towards virtual populations for human efficacy prediction in lung cancer: preclinical to clinical translation of anti-PD-(L)1 treatments"
Nataly Kravchenko-Balasha
(The Institute of Biomedical and Oral Research, The Hebrew University of Jerusalem, Israel, Israel)"Computational quantification and characterization of independently evolving cellular subpopulations within tumors is critical to inhibit anti-cancer therapy resistance"
Alexander R. A. Anderson
(Integrated Mathematical Oncology Department H. Lee Moffitt Cancer Center & Research Institute, USA)"Exploiting evolution to design better cancer therapies"
Andrew Gentles
(Departments of Medicine and Biomedical Informatics, Stanford University, USA)"Building an atlas of cell states and cellular ecosystems across human solid tumors"
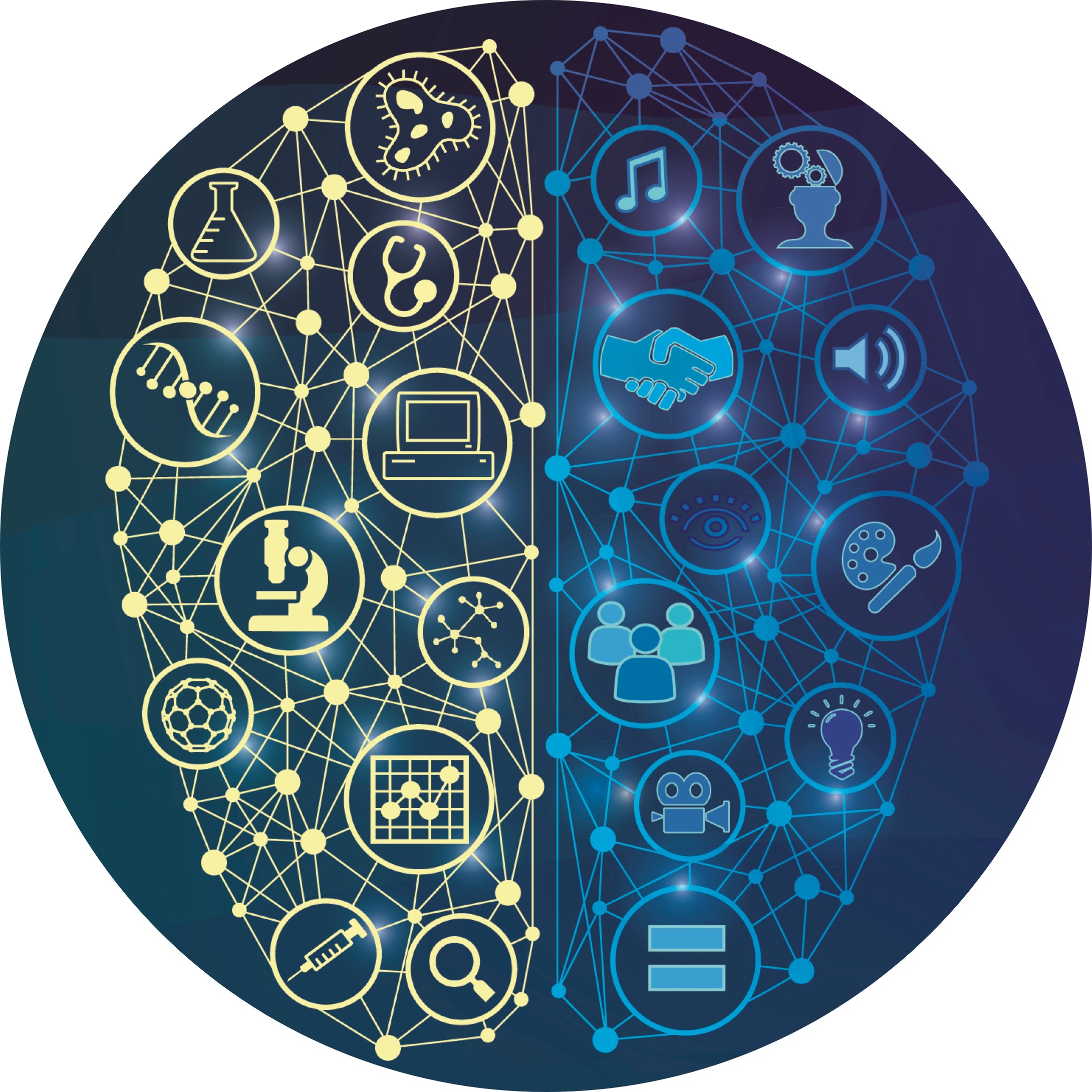