MFBM-MS06
Mathematical and computational methods to augment the reliability of biological models for better decision-making
Tuesday, June 15 at 04:15am (PDT)Tuesday, June 15 at 12:15pm (BST)Tuesday, June 15 08:15pm (KST)
Organizers:
Vincent Lemaire (Genentech, CA, USA, United States), Khamir Mehta (Amgen, Inc, United States), Malidi Ahamadi (Amgen, CA, United States)
Description:
Mechanistic quantitative systems pharmacology (QSP) models are often limited in their utility by the lack of data for complete characterization of the model parameters and the associated parameter uncertainty. Application of such models in drug development will benefit from robust qualification of the model in terms of parameter estimates and also creation of a representative ‘virtual population’ that will likely reflect the observed variability in biological outcomes. Various methodologies have been employed to achieve this goal; including virtual populations-based methods, model reduction to enable direct estimation of parameters, its variability and uncertainty, as well as model averaging to enable spanning across different model functions for individual model components. Recently, data analytics and machine learning methods have also been employed in conjunction with QSP models to expand the utility of complex models, but also to help in the model building process. This session will highlight the key strengths and weaknesses of the above methods as applicable to specific scenarios in the drug development process. Importantly, it will also reflect on the outstanding challenges in the area and will pave the way to further discussions and pollination of ideas and techniques to overcome them.
Chris Rackauckas
(MIT and Pumas AI, MA, USA, United States)"Accelerating Quantitative Systems Pharmacology with Machine Learning"
Oleg Demin Jr
(InSysBio, Russia)"Implementation of variability or uncertainty in parameter values to validate QSP models."
Gianluca Selvaggio
(Fondazione The Microsoft Research - University of Trento Centre for Computational and Systems Biology (COSBI), Italy)"Parameter free approaches in QSP: modelling the cytokine release following bispecific T-cell engager therapy"
Sietse Braakman
(AbbVie Inc., Quantitative Translational Modeling Group, United States)"A framework for the evaluation of QSP models, with a focus on verification, validation and uncertainty quantification (VVUQ) methods"
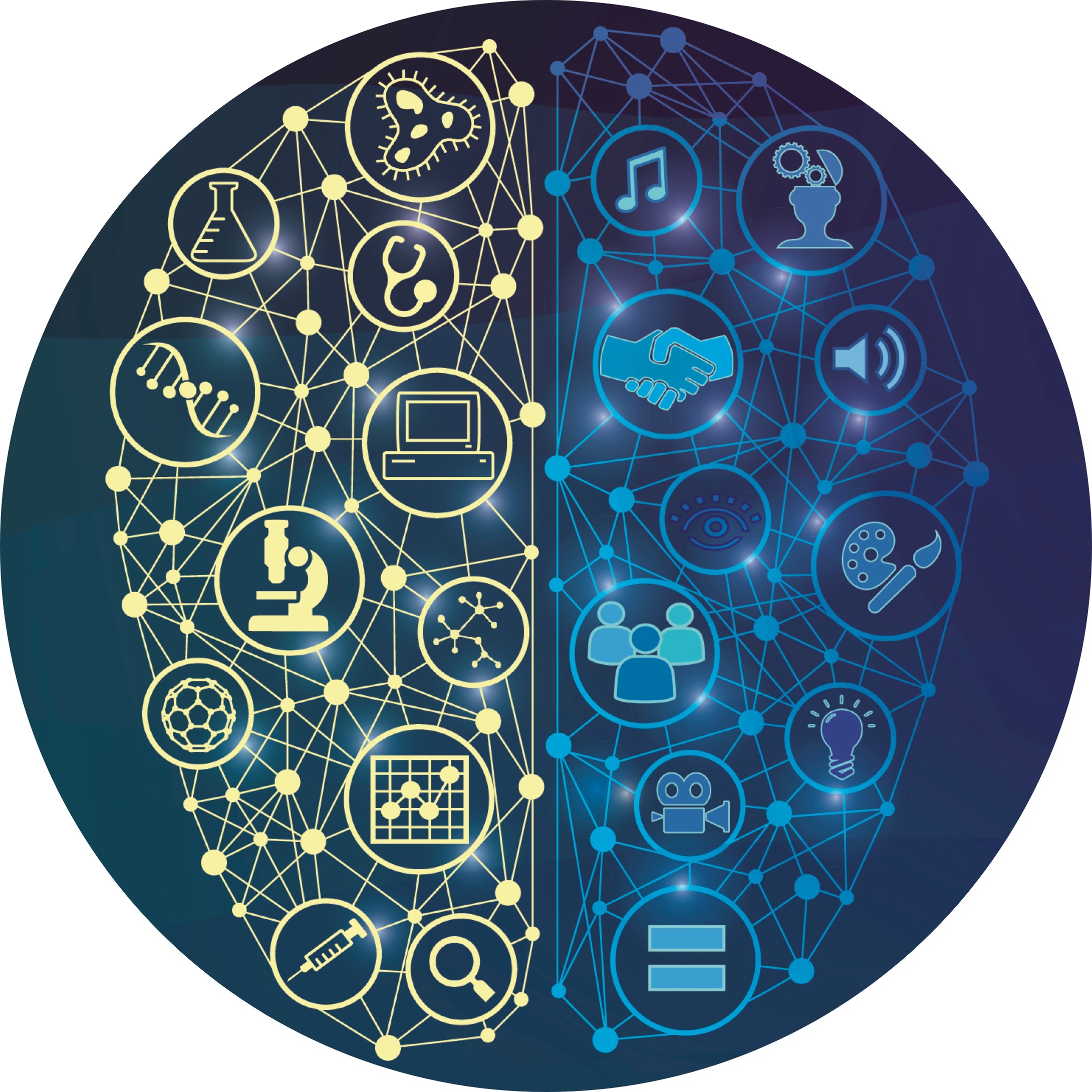