ONCO-MS01
Mathematical approaches to advance clinical studies in oncology
Monday, June 14 at 09:30am (PDT)Monday, June 14 at 05:30pm (BST)Tuesday, June 15 01:30am (KST)
Organizers:
Heyrim Cho (University of California Riverside, USA), Russell Rockne (City of Hope Comprehensive Cancer Center, USA)
Description:
In this session, we bring together researchers in mathematical oncology to discuss methodologies for translational research in oncology. The advance of clinical data acquisition technologies, such as genome sequencers and new imaging techniques, are providing new opportunities in mathematical oncology. Mathematical modeling and quantitative framework can effectively leverage clinical data to describe various aspects of cancer progression and drug response. Moreover, models calibrated to individual patient data can predict treatment outcome and help design personalized treatment regarding the drug combination, dosage, and scheduling to improve treatment outcome. Here we highlight several applications of mathematical modeling to clinical oncology, including analysis of clinical data and clinical trials designed with mathematical modeling.
Hitesh Mistry
(University of Manchester, UK)"Complexity/Simplicity of Oncology Pharmacodynamic Markers/Mathematical Models in the Clinic versus Drug Development"
Renee Brady
(H. Lee Moffitt Cancer Center and Research Institute, USA)"Predicting Response to Adaptive Therapy in Metastatic Prostate Cancer Using Prostate-Specific Antigen Dynamics"
Aleksandra Karolak
(City of Hope Comprehensive Cancer Center, USA)"A Quantitative Systems Pharmacology Model to Improve Graft Versus Host Disease Outcomes"
Kit Curtius
(University of California San Diego, USA)"Predicting Risk of Progression to Advanced Neoplasia in Patients with Ulcerative Colitis"
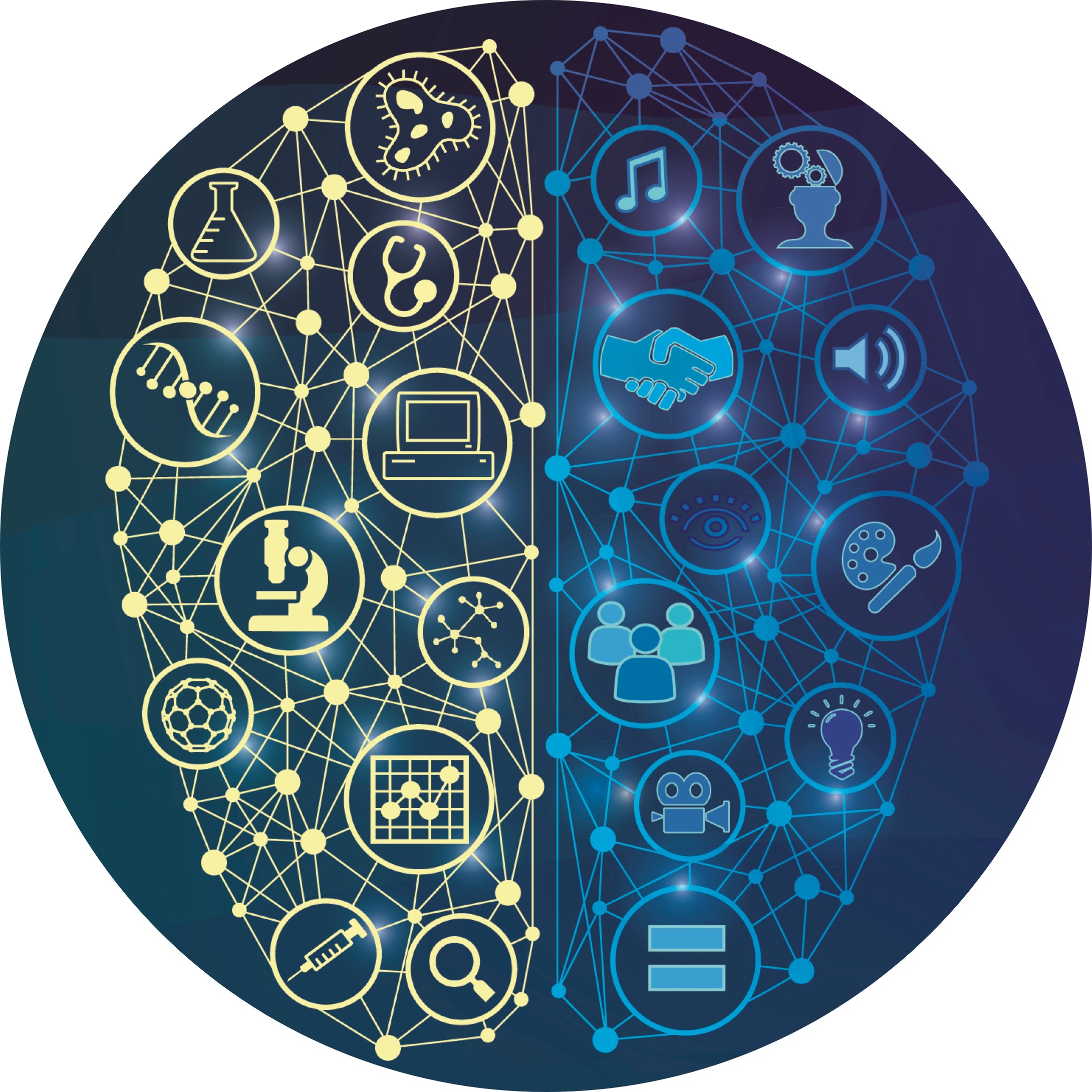