MEPI-CT01
MEPI Subgroup Contributed Talks
Monday, June 14 at 03:15pm (PDT)Monday, June 14 at 11:15pm (BST)Tuesday, June 15 07:15am (KST)
Share this
Michael Irvine
Simon Fraser University"Quantifying transmissibility of COVID-19 and the impact of intervention in long-term care facilities"
Carlo Davila-Payan
Centers for Disease Control and Prevention"Analysis of the yearly transition function in measles disease modeling"
Luis Manuel Munoz-Nava
Center for Research and Advanced Studies"‘Learning Bubbles’ are an effective and safe alternative to schools reopening during the COVID-19 pandemic"
Glenn Ledder
University of Nebraska-Lincoln"A Model for COVID-19 with Limited Vaccination"
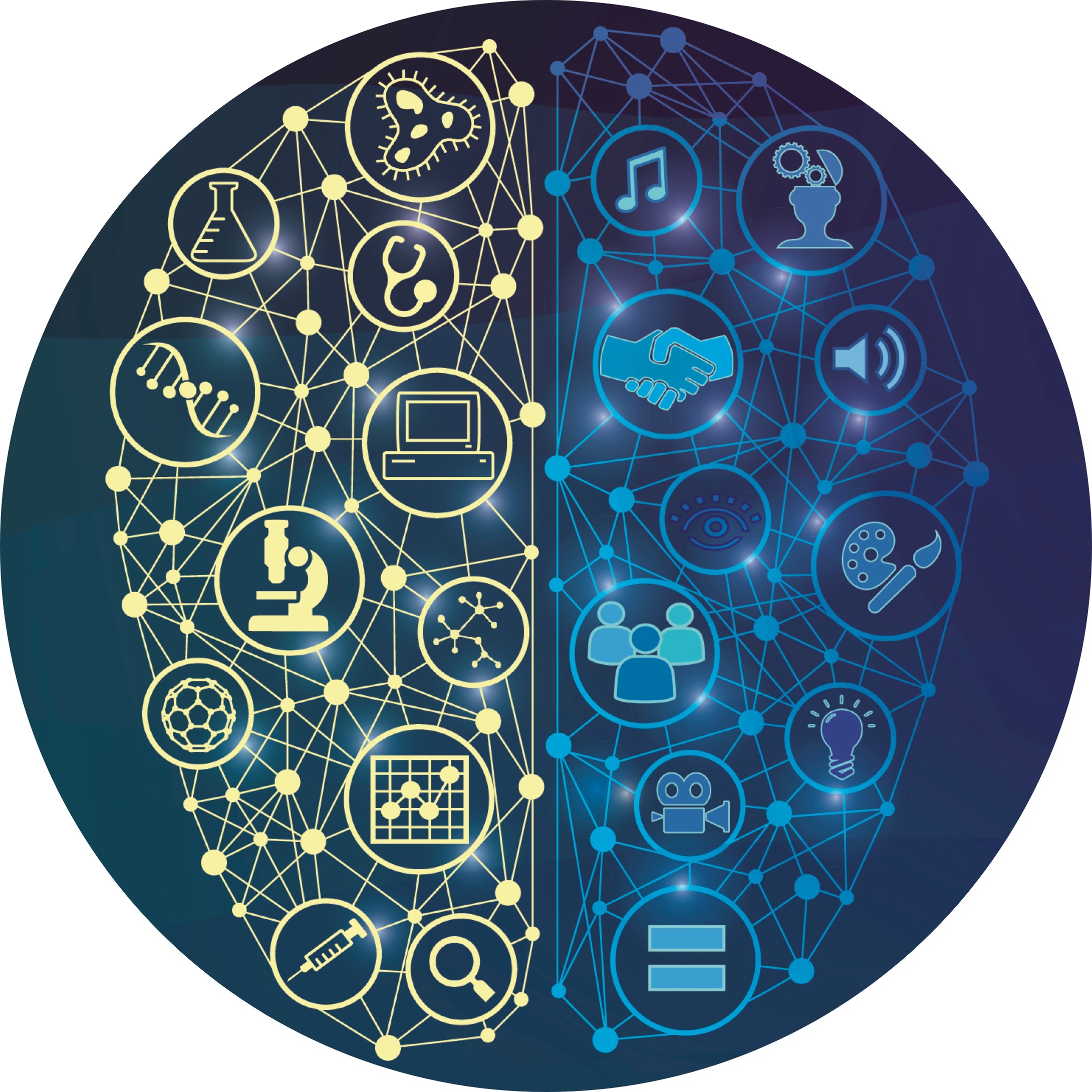