DDMB-MS09
Machine Learning and Data Science Approaches in Mathematical Biology: Recent Advances and Emerging Topics
Tuesday, June 15 at 05:45pm (PDT)Wednesday, June 16 at 01:45am (BST)Wednesday, June 16 09:45am (KST)
Organizers:
Paul Atzberger (University of California Santa Barbara, USA), Smita Krishnaswamy (Yale University, USA), Kevin Lin (University of Arizona, USA)
Description:
Biological investigations have resulted historically in the development of many new methods for data analysis. This session aims to discuss recent advances both concerning new biological application areas and algorithms drawing on increasingly large datasets and availability of computational resources. Topic areas include but are not limited to, data-driven modeling, applications of deep learning to problems in biology (sequence analysis, protein folding, experimental design, control), physics-informed machine learning, kernel methods for biological systems, linear and non-linear system identification, hybrid data-driven simulation methods, and other areas. The session also aims to facilitate discussions on emerging methods and areas for the biological sciences where data analysis is playing increasingly central roles.
Zhuo-Cheng Xiao
(Courant Institute, NYU)"A data-informed mean-field approach to mapping cortical landscapes"
Andrea Arnold
(Worcester Polytechnic Institute, USA)"Data Assimilation for Time-Varying Parameter Estimation in Biological Systems"
John Fricks
(Arizona State University, USA)"A Bayesian Analysis of 2-D Motor-Cargo Complex Dynamics"
Mengyang Gu
(University of California, Santa Barbara, USA)"Uncertainty quantification and estimation in differential dynamic microscopy for biomaterials characterization"
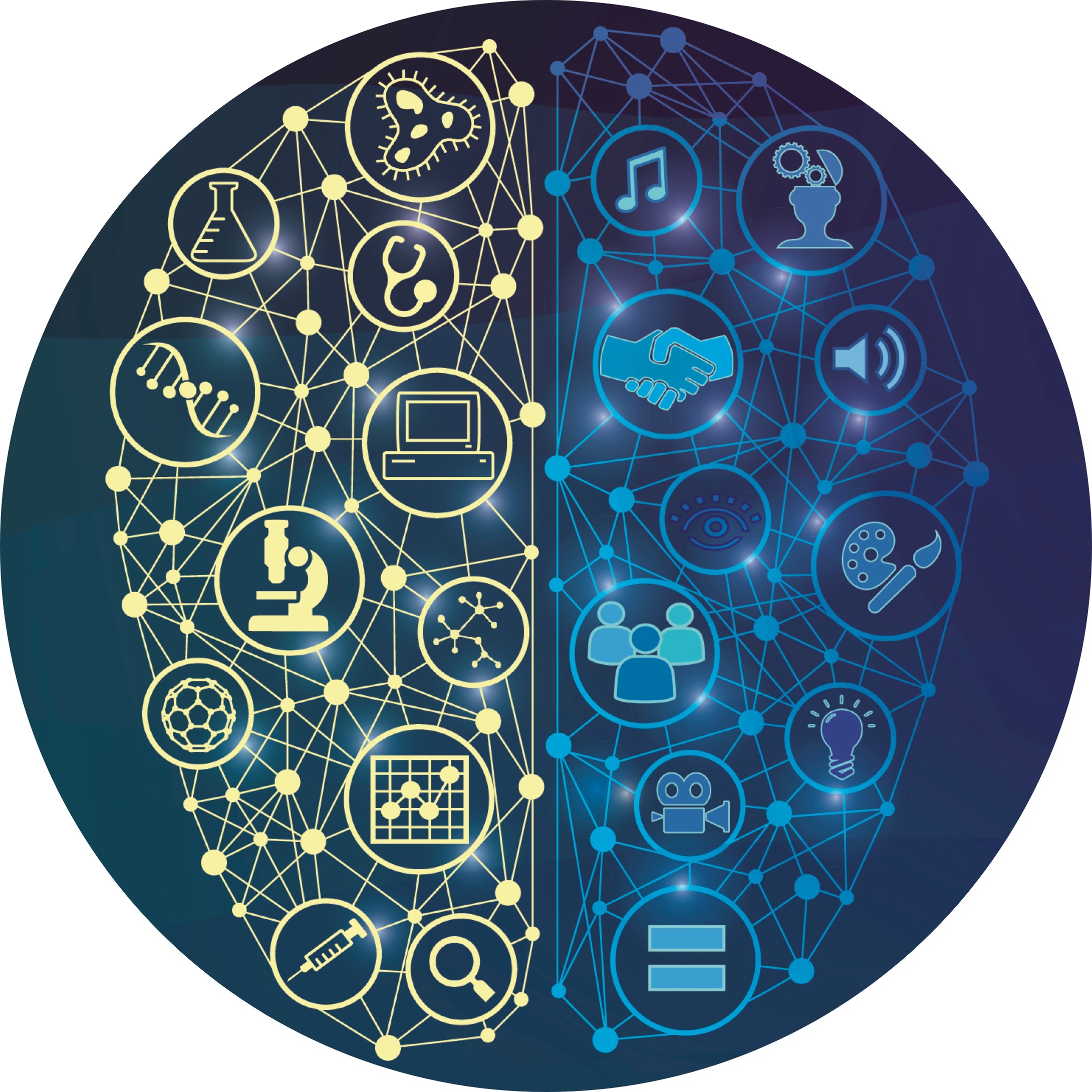